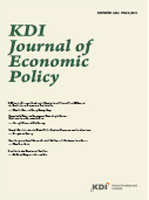
- P-ISSN 2586-2995
- E-ISSN 2586-4130
The Korean government has provided various policy devices to boost technology transfers between academia and industry since the establishment of the Technology Transfer Promotion Act in 2000. Along with the enactment of the law, the Korean government mandated the establishment of a technology transfer office at national and public universities and encouraged technology transfer activities. Despite the quantitative expansion of technology transfer offices (TTOs), operational inefficiency was brought up. As a supplementary policy, the Korean government implemented a line of projects to support the labor and business expenses of leading TTOs. This research questions whether the project greatly affected the technical efficiency of TTOs. We analyze publicly available university panel data from 2007 to 2015 using a one-step stochastic frontier analysis. The results suggest that the program was marginally effective at shifting the technical efficiency distribution to the right on average, but it failed to maximize its impact by diversifying the policy means based on targets. The marginal effects of the program on technical efficiency differ according to the research capability and size of each school. We also compare technical efficiency against the licensing income at the start and end of the program. Technical efficiency increased for the leading TTOs, and both measures show improvements for unsupported TTOs. Our empirical results imply that diversifying the program for universities with different characteristics may have improved the effectiveness of the policy.
Technology Transfer, Technology Transfer Office, Efficiency, Stochastic Frontier Analysis
L24, O32, O38, I28
The assessment that the Bayh–Dole Act positively affected the commercialization of publicly funded research has led to the implementation of similar policies in many countries. A few policies focus on legal reformations related to the ownership of intellectual property rights produced by publicly funded research to facilitate commercialization. Another set of policies concerns the establishment or fostering of intermediaries for the technology transfer functions of universities. Several researchers were critical of these policies and skeptical about their effectiveness. Mowery and Sampat (2005) argued that the success of Bayh–Dole is actually due to universities' efforts to engage in university-industry collaboration and technology transfers even before the enactment of the law and that these efforts are rooted in the scale and structure of the U.S. higher education system. They predicted that such efforts to emulate the Bayh–Dole policy will not achieve great success. Indeed, industry–academia cooperation in Western European countries, Japan, and Korea still tends to be led by the respective governments, and an atmosphere of autonomous cooperation with the private sector is not easily formed.
Nonetheless, due to the increasing complexity and diversity of technological advances, voluntary activities for knowledge spillover by the private sector are required, and governments continue to make efforts to create ecosystems in which such activities can thrive. In countries without a lengthy history of university–industry collaboration, such as the United States, various policy interventions have attempted to achieve smooth transfers of public research outcomes. The Korean government's technology transfer policies have also been part of the efforts. The Korean government has provided various policy devices to boost technology transfers between academia and industry since the establishment of the Technology Transfer Promotion Act (TTPA) in 2000 and the Industrial Education Enhancement and Industry-Academia Research Cooperation Promotion Act in 2003.1
Despite the efforts of the Korean Government, university—industry cooperation is not considered active. In particular, technology transfer offices (TTO), which are responsible for patents, licensing, and commercialization, are still judged to lack expertise and competence, despite the fact that they are legally required to be established.2 As the government led the establishment of TTOs at national and public universities, the expansion of university TTOs has been more quantitative within a short period. However, TTOs suffered from operational inefficiency because most of them did not have enough experience and/or specialists such as patent attorneys and technology valuation specialists. These problems are not easily solved because the corresponding universities have not provided sufficient funding for the operation of the TTOs. The government recognized the structural problems and attempted to enhance the capabilities of TTOs through supplementary financial support. For this reason, starting in 2006 the government began to implement the financial support project known as the Leading TTO Cultivation Project to subsidize the labor and business expenses of TTOs which have shown relatively high performance capabilities.
At the moment when the Leading TTO Cultivation Project, which was originally planned for five years, was extended for another five years, the TTOs of Korean universities were evaluated to have better competence in terms of the number of experts, technology transfer income, and patent registrations. However, it is not known how much of the improvement is due to the policy. The purpose of this paper is quantitatively to evaluate the effects of the policy and present future policy directions.
Technology transfer is a topic studied from a wide variety of aspects. Bozeman (2000) and Bozeman et al. (2015) found several major directions in the broader technology transfer literature under the dimensions of the contingent effectiveness model. In particular, Bozeman et al. (2015) found that the discussion of recent studies is centered on university settings. While many studies focus on the impact and effectiveness of technology transfers, factors affecting the effectiveness of technology transfers are still being explored (Caldera and Debande, 2010). As technology transfers involve many actors and the final outputs of TTOs vary according to the institutional goal, various determinants are being studied. Although the output of TTOs is not singular but rather mixed, the number of technology transfers, royalty income, and licensing fees are recognized as the main outputs (Thursby and Kemp, 2002; Carlsson and Fridh, 2002; Siegel et al., 2007). Often, intellectual property rights ownership, start-ups, or spin-offs based on technologies invented in university labs are considered to be the outputs of TTOs (Thursby and Kemp, 2002; Carlsson and Fridh, 2002; Friedman and Silberman, 2003).
A large portion of the studies on technology transfers focus on finding the determinants of technology transfers, and many factors have been explored in different countries. Listing each factor is difficult because previous studies identify many determinants of a technology transfer. One of the reasons for the various and complicated determinants is that technology transfers are complex activities that involve multiple stages. Defining a typical production function as one for the manufacturing sector is challenging. Various determinants can be categorized as the primary inputs for invention, secondary inputs for technology transfers, and other environmental factors. Interestingly, the outputs from technology production are actually the important intermediate inputs for the stage of the technology transfer. Another portion of studies attempts to estimate the production function and productivity of technology transfer units, which are TTOs in most cases (Siegel et al., 2003; Thursby and Kemp, 2002; Thursby and Thursby, 2002). These studies seek to find the determinants of the improved technical efficiency of technology transfer units.
Several countries have implemented technology transfer policies under government initiatives, but few empirical studies have examined how these policies affect technology transfers. Goldfarb and Henrekson (2003) showed that policy efficiency can change according to the policy delivery structure by comparing cases in Sweden and the United States. In particular, they argued that the top-down nature of Swedish policies may be an obstacle to the commercialization of academic achievements, whereas competition between universities and researchers for research funding has allowed academics to interact with industry actively. The lack of empirical research on the effectiveness of policies may be due to the failure to find a policy environment that influences technology transfers independently in many countries. A major contribution of the present study lies in identifying the policy environment and conducting an empirical analysis to confirm the effectiveness of a policy.
In this study, we analyze the effects of the Leading TTO Cultivation Project implemented by the Korean government from 2011 to 2015 on the technical efficiency of university TTOs. In particular, we utilize a one-step stochastic frontier analysis with publicly available university panel data of Korea from 2007 to 2015. The main finding is that the TTO policy effectively improved the technical efficiency of university TTOs on the average, whereas the effects of the policy could be improved if it were fine-tuned according to the characteristics of the TTOs and universities.
The remaining parts of the paper are organized as follows. In the next section, we introduce the technology transfer policy in Korea and the financial support program for TTOs, which is the main policy instrument analyzed in section III. In section III, we analyze the impacts of the TTO support program by using a one-step stochastic frontier analysis. We review the one-step stochastic frontier model suggested by Wang and Schmidt (2002), and illustrate the results from an empirical analysis. Finally, section IV concludes the paper.
To facilitate exchanges of knowledge and technology between science and technology academia and industry, the Korean government has enacted laws and implemented various plans and support projects accordingly. Korea had emphasized linkages between industry and academia during the process of industrialization in the 1960s, but only in a few cases were the research outcomes of public research institutes (PRIs) transferred or put into practical use by the end of the 1990s. The TTPA was enacted in 2000 to promote the development of science and technology and the commercialization of achievements in these areas. The Science and Technology Innovation Office3 began to manage and utilize research results with government support starting in 2005. In 2006, the TTPA was amended to increase the incentives for the technology commercialization of PRIs. In addition, the Korean government has enacted various laws for the protection of intellectual property rights, the promotion of university–industry collaboration, support for the development of new technology, the creation of a proper ecosystem, and participation by private companies.
Along with such legal assistance, the Technology Transfer and Commercialization Plan (TTCP) was implemented in 2001. The plan aims to integrate the management of the details of various ministries involved in technology transfer and commercialization activities. At the beginning, the plan focused on building infrastructures (e.g., the technology trading market, National Technology Bank) and establishing intermediary organizations such as an industry–academia cooperation foundation, TTOs, and technology evaluation institutions. In the second phase of the plan, the emphasis was on building a system for technologically innovative businesses by allowing technology in-kind contributions and expanding technology finance. Owing to these efforts, social awareness of technology transfers and commercialization spread in a short period and a quantitative expansion was possible. However, the lack of links between detailed projects and a policy that prioritizes quantitative achievements were obstacles to smooth collaboration between researchers and industry. To solve these problems, the plan focused on establishing a technology-oriented ecosystem and encouraging voluntary participation by the private sector. To this end, the role of the technology transfer intermediaries has been to attract attention, and the organizational expertise of TTO has been incorporated into the detailed goals of the plan since 2012.
The Korean government's technology transfer policy has expanded quantitatively with the TTCP, but these efforts have generally resulted in projects targeting SMEs. Relatively little attention has been paid to universities, even if policy programs that qualify for universities and their subordinate organizations actually require company participation or are intended to help SMEs that are technology consumers. In other words, only few policies have attempted to solve the problems of university TTOs on the side of the technology provider. Technology transfer policies have been centered on enterprises because policy authorities took the existence of intermediary organizations for granted and did not recognize that the intermediaries had not been developed sufficiently.
The Leading TTO Cultivation Project is one of the few policies aimed at university TTOs. The project was actually initiated by the Small and Medium Business Administration in 2001 and continued on a very small scale until 2006. In 2006, the Ministry of Knowledge Economy and the Ministry of Education, Science, and Technology expanded this project and started a new project called Connect Korea, benchmarking the CONNECT program of the University of California, San Diego (UCSD). The project was planned as a five-year project, three years to complete the first half and two years for the second half, and 18 universities and their TTOs were supported by the project. At its beginning, TTOs were still being established in many universities, implying that this project contributed to the spreading of TTOs throughout the country and to expanding professional employment (Table 1) rather than contributing to the actual growth of the TTOs.
Note: 1) 1Patent Attorneys; 2Technology Transfer Agents; 3Certified Valuation Analysts, 2) Figures are the workforce of 18 universities which participated in the Connect Korea program. The Connect Korea program ran from 2001 to 2005.
In 2010, when the project ended, Korean universities were still in a poor situation. TTOs are operated as subordinate organizations of the University–Industry Collaboration Foundation, which is responsible for more complex tasks. Hence, concentrating on technology transfers was difficult, and only four or five staff members were involved. Although the number of experts increased, this was limited to universities with the proper financial leeway.
After the end of the project, the government expanded its scale and implemented a new project termed the Leading TTO Cultivation Project from 2011 onwards. A major difference from the previous support project is that TTOs with superior performance capabilities are prioritized while second-best TTO groups are supported by a type of consortium. TTOs are divided into two types according to their existing technology transfer performances and their capacities. At the time of the project in 2011, leading TTOs received KRW 150 million to KRW 300 million, and the TTOs of the consortium received KRW 50 million. This project was also planned as a five-year project, with two years allocated for the first half and three years for the second half. In total, 24 TTOs were supported in the first half of the project and 30 TTOs were supported in the second half. The subsidies were designated to be used to cover labor costs, technology discovery, evaluations, and marketing. The project also provided educational programs for technology transfers, but this was not the main part. Essentially, the project was a simple program to support the operating expenses of TTOs.
In the next section, we examine the impact of the Leading TTO Cultivation Project on efficiency improvements at TTOs through an empirical analysis.
The stochastic frontier analysis (SFA) and the data envelopment analysis (DEA) methods are widely used methodologies which analyze how efficient decision-making units are. The SFA is based on the idea that no economic unit can exceed a theoretical production frontier; therefore, the degree of inefficiency can be estimated by the gap between the ideal production frontier and the productivity of each economic unit. The main goal of the conventional SFA is an empirical estimation of the relative inefficiency (or efficiency) of individual economic units compared to the best practice unit.
A simple stochastic frontier model can be written as follows:
Eq. (1) is a log-transformation of a Cobb–Douglas production function. The empirical literature on the production function generally seeks to estimate the parameters of the production function that passes through the middle of data points. Arguments in the stochastic frontier literature redirected attention from the production function to deviations from that function (Greene, 2008). Generally, SFA focuses on estimations of the deviation from the production frontier with the assumption that the error term includes unobserved productivity, which is additive to the white noise. The error term εit can then be decomposed into the unobservable inefficiency term uit and white noise vit. If an economic unit, for instance i = m , is producing on the production frontier, then its inefficiency is zero by definition; i.e., umt = 0 , whereas the inefficiencies of other economic units are greater than zero; i.e., uit > 0 , where i ≠ m . In other words, a one-sided distribution can be assumed for the inefficiency term uit . Under this assumption, the relative inefficiency is comparable by estimating the inefficiency distribution and parameters of the production function using, in this case, Eq. (1).
Although efficiency4 is essentially unobservable, we can think of the sources of efficiency. The early literature on the sources of efficiency constructed what was termed an efficiency equation using the estimated efficiency measure shown in Eq. (1) as a dependent variable, estimating it separately. These studies, using what is known as the two-step approach, consider efficiency factors exogenous to the independent variables of Eq. (1).
However, the endogeneity issue is prevalent as long as we cannot control the relevant variables precisely, and the estimates are biased when we ignore the endogeneity problem. For this reason, a set of studies after Kumbhakar et al. (1991) stressed the usefulness of the one-step approach, which estimates the system of the production function and the efficiency equation at the same time. In particular, Wang (2002) and Wang and Schmidt (2002) showed that estimates from two-step approach can be biased, with the following reasoning. First, there is a possibility of a correlation between the explanatory variables for the production function and the determinants for the efficiency equation. Second, it is challenging to exclude the possibility that omitted variables exist in the efficiency model used in the second step. Third, it is highly probable that the estimated efficiency in the first step without considering the determinants of efficiency is downwardly biased.
A simple means of introducing influences in the inefficiency model is to consider the location and scale of the distribution. Studies such as those by Kumbhakar et al. (1991), Huang and Liu (1994), and Battese and Coelli (1995) proposed the parametrization of the mean of the pre-truncated inefficiency distribution. As an extension, Caudill and Ford (1993) and Caudill et al. (1995) put forward the parameterization of the mean and variance of the pre-truncated inefficiency distribution to allow heteroscedasticity in uit and/or vit . Additionally, Wang (2002) introduced a methodology to allow non-monotonic efficiency effects owing to the possibility that the effects of determinants on the efficiency distribution are not monotonic. For example, the accumulation of experience by farmers can enhance production efficiency even if the marginal effect of age on efficiency is negative.
In the present study, we apply the methodology suggested by Wang (2002) to estimate the marginal effects of policy intervention on the technical efficiency of university technology transfer offices. The main model is expressed in the following system of equations,
where zit denotes the vector of the determinants of inefficiency. As noted above, Eq. (2) represents
a log-transformed production function, and Eq. (3) is the composed error of the inefficiency
and white noise. We assume that the white noise follows a normal distribution with
zero mean and variance of . Inefficiency, uit , is assumed to have a truncated normal distribution, 5 whose pre-truncated distribution has a mean of μit and variance of
. Following Wang (2002), we parametrize both the mean and variance of the pre-truncated efficiency distribution,
as expressed in Eq. (6) and Eq. (7).
Given that μit and represent the mean and variance of the pre-truncated distribution, respectively,
δ and γ are not precise measures of the effects of zit on the efficiency distribution. Instead, Wang (2002) provided the functional form of the marginal effects of each determinant on the location
of the efficiency distribution, as follows6:
Here, z[k] denotes the k th element of the determinants, Λ = μit / σit , and ϕ and Φ represent the probability density function and cumulative distribution function of the standard normal, respectively.
The data sources of this paper are three fold. The primary data source is the Information Service of Higher Education (ISHE) in Korea. It provides publicly available information and regulated relevant details of all universities in Korea. The variables we collected from this source include operational details of the office of research affairs, research activities and funding, and general information pertaining to universities. We also collect the number of patents owned by each university from the Worldwide Patent Statistical Database (PATSTAT) of the European Patent Office and the Korea Institute of Patent Information (KIPI). Finally, we obtain information about the recipients of government financial support programs from business description materials held by the Ministry of Science, ICT, and Future Planning (MSIP) and the Ministry of Education (MOE).
The number of technology transfers and royalty income are collected on the basis of the information reported by the university–industry cooperation foundations based on the contracts for technology transfers. In this case, technology transfers are limited to cases in which the developed technology is purchased or licensed, and transfers of technology that has not been developed, such as technical consultations, industrial joint research, and personnel exchanges, are excluded. Royalty income refers to the amount of money actually deposited in the survey year, regardless of the contract year, excluding VAT, and the number of contracts means the number of contracts for technology transfers made in the survey year. The operating expenses for university–industry cooperation were collected based on closed accounts data. This variable refers to expenditures on operating expenses for industry-academia cooperation among the accounting categories of the Industry-Academia Collaboration Foundation. This item is composed of industry-academy cooperation research funds, educational administration fees, intellectual property rights operation and transfer fees, school facility fees, industry-university cooperation rewards, and other industry-academic cooperation fees.7 Next, research funds include only cash in the amounts agreed upon during the base year. Among these, the amounts for government subsidies are research funds supported by the central government and ministries and not local governments. Total financial support means grants through financial support projects. A financial support project refers to a project that is managed through an on-campus institution and that meets one of the following conditions: improvement of educational conditions, development and operation of the curriculum, improvement of the undergraduate system, cooperation between industry and academia, and research and development by professors and students.
The range of data is nine years, from 2007 to 2015 inclusive. The number of institutions of higher education in Korea has gradually increased.8 Such institutions exist in various forms and pursue different purposes. Some do not aim to transfer technology, or this activity is impossible because they do not have technologies to sell. Therefore, we limit the sample to universities that are capable of technology transfers. We exclude universities that did not have technology transfer revenue for the sample period. In addition, universities within the lower 10% in terms of average royalty income for the three years between 2013 and 2015 inclusive are excluded. The final sample collected on this basis is an unbalanced panel with 127 observations per year. All nominal variables are adjusted to real variables with a GDP deflator for 2010. The summary statistics are displayed in Table 2, and the correlation coefficients between the variables are given in Table A1 in the Appendix.
Note: The data source for TTO Operational Details, Research Activities, and General Information is ISHE. Domestic and Foreign Patents are from KIPI and EPO PATSTAT, respectively. All monetary variables are in KRW. Units of Operating Expenses, Total Research Funding, Government / Private / Local Research Funding, Total Financial Support, and Total Education Expenses are in billions of KRW and Royalty Income is in millions of KRW in this table.
As we have discussed in Section I, there is only a rough consensus on the outputs and inputs of the TTOs' production function. Although TTOs aim to support researchers' patent activities and ultimately to increase the number of patents owned by universities, it is also an important operational goal to increase technology transfer income by selling patents already owned. For this reason, one may regard patents as a part of the outcome that TTOs produce. However, the contributions of TTOs during this process are not research activities for patent production but rather overall an auxiliary role for the registration and management of IP. Naturally, we consider IP as an intermediate input for TTOs to create technology transfer outcomes. In this study, we assume that the intellectual property rights of each university are intermediate inputs that can be utilized by TTOs.
To sum up, the number of technology transfer contracts and the amounts of royalty income are considered as outputs, and inputs consist of operating expenses of TTOs, the number of TTO employees and the cumulative numbers of domestic and foreign patents owned by the affiliate university. The other variables include a policy dummy and control variables describing the university research environments. The policy dummy indicates a value of 1 for all years i in which the TTO policy subsidizes university j, and 0 otherwise. The control variables for university research environments include the size of the university as measured by the funding amount, the number of faculty members, the number of students, or total education expenses, the size of the TTO proxied by operating expenses, and other variables to control for the general characteristics of universities, such as the location of the university or whether a university is private or public.
With these variables, we estimated the system of equations, Eq. (2) to Eq. (7), by means of maximum likelihood estimations, as in Battese and Coelli (1995). All variables but dummy variables are logarithmic, and lagged values of the TTO policy dummy, operating expenses, are used. Because there is a possibility of a sample selection problem with regard to the TTO policy dummy, we used the estimated inverse Mill’s ratio from a probit model9 for the TTO policy dummy.
Table 3 illustrates the parameter estimates. Table 3 consists of the estimation results of Frontier Equation Eq. (2) and Inefficiency Equation Eq. (5). The table shows the results from seven specifications. We keep the input variables for the Frontier Equation unchanged, whereas the specification for the Inefficiency Equation varies. We take logarithm of the output and input variables; therefore, the estimated coefficients for the Frontier Equation can be interpreted as the output elasticities of each input factor. We assume that there is no exogenous variable that affects the efficiency of the TTO operation in specification (1), which is our baseline model. In this case, the output elasticities of all variables but operating expenses are significant and positive. The output elasticity of domestic patents granted is the largest, whereas that of foreign patents granted is the smallest. The coefficient estimates of the Frontier Equation are stable across specifications; therefore, the estimation of the Frontier Equation is robust for this model.
Note: 1) Numbers in parentheses are Huber-White Robust Standard Errors, 2) *p<.1, **p<.05, ***p<.01.
We set μit as a function of the TTO policy in specification (2). The magnitudes of the coefficient estimates of the Frontier Equation do not change much, and the coefficient for operating expenses is estimated to be positive and significant. The result revealed that the effect of the TTO policy on the mean of the inefficiency distribution is negative but statistically insignificant. For specifications (1) and (2), the constant term is estimated as negative but insignificant. This implies that inefficiencies are likely to be distributed near zero, which is ideal but unrealistic. In specification (3), we added the number of faculty members, which is a proxy for research capacity, as a determinant of inefficiency. Along with the TTO policy variable, the number of professors has a negative and significant effect on the mean of the inefficiency distribution. In other words, the efficiency of TTO improves distributionally as the number of professors increases or if a TTO is supported by a governmental financial support policy.
In specification (4), we added a public dummy that indicates whether the universities to which a TTO belongs are public or private. Given that the estimates from this specification are comparable to those from specification (3) and the coefficient estimate of the public dummy is insignificant, it can be concluded that the university type does not affect the efficiency of the TTO.
Specifications (5)-(7) include two funding variables as inefficiency determinants: Research Funding from various sources and Total Financial Support. When research funding for each source is included in (5), only government research funding reduces inefficiency significantly. The magnitude of the impact of government research funding in (5) is comparable to that of total research funding in (6). This is reasonable considering that nearly 71% of total research funding comes from the government for universities in Korea. The results from specifications (6) and (7) indicate that either total research funding or total financial support improves the efficiency of TTOs.
Because specifications (1)-(4) are nested into specification (5), we perform likelihood ratio tests on the hypotheses that nested models are preferred in specification (5). The results suggest that specifications (1)-(4) are rejected in favor of specification (5) at the 5% level.
It should be noted that the estimates displayed in Table 3 are not the marginal effects of determinants on the mean of the inefficiency distribution, E(uit) despite the fact that the signs coincide. In Eq. (6), μit is not the mean of inefficiency; rather, it is the mean of the pre-truncation of the inefficiency distribution because we assume that the distribution of inefficiency takes a half-normal form. The marginal effects of determinants on the mean of the inefficiency distribution can be expressed by Eq. (8) from Wang (2002). The marginal effects can be derived for each of the observations.
In Table 4, the sample mean of the marginal effects are listed. The negative sign of the estimated marginal effect means that the inefficiency is alleviated on average as the corresponding determinants become larger. One can find that all but the public university dummy have negative marginal effects on mean inefficiency. In addition, the TTO policy has the greatest marginal effects, indicating that the TTO policy played an important role in reducing the operational inefficiency of TTOs on average.
Note: Numbers in parentheses are 1,000 bootstrap standard errors.
Figure 1 and 2 illustrate the observation-wise marginal effects of the inefficiency determinants. The horizontal axis represents the determinant and the vertical axis is the marginal effect. Figure 1 is generated from specification (5), as is shows the largest log-pseudolikelihood.10 First, Panel (a) shows the differences in the marginal effects of the TTO policy by support type. As discussed in Section II, the financial support program for TTOs was implemented and aimed at two groups: leader groups and consortia. As indicated by the policy name, this policy was designed for leading TTOs, but it also provided an opportunity for the remaining TTOs for which the performance levels were second-best. Support for this non-leader group was provided in the form of a consortium, and eight universities were selected for the program. From Panel (a), the marginal effects of both types are skewed towards the larger side, which means that most of the TTOs have small marginal effects, and only a few TTOs enjoy relative greater improvements in efficiency by the policy. The horizontal line in the box plot represents the median of the distribution. We can find that the median of the leader group is higher than that of the consortium, which demonstrates that the marginal improvement of efficiency in the consortium is greater when TTOs are supported by the financial support policy. Note that the variance of the marginal effects in the leader group is smaller than that in the consortium. This provides a rationale for the need to investigate how the effects of the policy differ with the characteristics of each university.
Panel (b) of Figure 1 shows the marginal effects of faculty size. We categorize faculty size into 100 units, and the largest category is the group of universities with more than 1800 faculty members. The results show that the marginal effects of faculty size are positive but non-monotonic. In particular, smaller universities are more likely to have a greater efficiency improvement than larger universities when the faculty size increases.
Note: The horizontal axis represents the determinant and the vertical axis is the marginal effect. The horizontal line in the box-whisker plot is the median and the dots are outliers. In panel (b), the unit of faculty size (horizontal axis) is one hundred and 18+ indicates a faculty size of more than 1,800 professors.
Figure 2 displays the marginal effects of different type of funding on TTOs’ technical efficiency levels, as estimated in specifications (5) – (7). The tendencies of the three panels are similar, as the three funding types are highly correlated. The leader group is indicated by the blue squares, the consortium is shown by the red triangles, and unsupported TTOs are denoted by the gray crosses. Mostly, the leader group tends to be larger in terms of how much funding they receive. Notably, a larger amount of funding received means a smaller marginal effect. Overall, the relationship between the marginal effects of funding and the funding size is positive and concave. However, the magnitude and variation in the marginal effects are much larger among unsupported TTOs.
Note: The observation-wise marginal effects of government R&D funding, total R&D funding, and total financial support are estimated in specifications (5), (6), and (7), respectively.
In sum, the results imply that the marginal effects of efficiency determinants are non-monotonic and increase as the research capacity of the affiliate university becomes smaller. Interestingly, the marginal effect of the TTO support policy is greater for TTOs supported in the consortium group. Taken together, the marginal effect of the TTO policy on mitigating inefficiency was greater for TTOs whose universities have less research funding and lower capacities. This result is somewhat out of line with the intent of the policy. We expect larger marginal effects for the leader group because the goal of the policy was to assist with the growth of TTOs which had shown better performance by supporting their operating and personnel expenses. The deviating policy effect is due to the uniform policy enforcement, which does not take into account the various characteristics of each university and/or TTO. Moreover, although many TTOs complain about difficulties due to a lack of professional manpower, there appears to be no relationship between the marginal effect of this policy and TTO employment. This implies that a policy that only supports personnel and business expenses does not solve the fundamental problem of TTO expert deficiency.
Finally, Figure 3 illustrates technical efficiency scores against royalty income in 2011 and 2015. The technical efficiency score can be derived from the estimated inefficiency, ûit , via −exp(ûit | εit) . In this figure, we can observe the change of each of the TTO’s positions. Royalty income is a measure for the final output of the TTO, whereas technical efficiency is a measure showing how efficiently it has operated through the ratio of input to output. As the TTO policy started in 2011 and ended in 2015, it is possible to check whether it has induced only external growth of the final output or caused an actual capacity enhancement of productivity or efficiency. In Figure 3, the vertical and horizontal lines for each panel are the averages of royalty income and technical efficiency, respectively. Although the average of royalty income did not change much, the average technical efficiency was improved. The efficiency level of the leader group has improved overall, while the variance in output (royalty income) was smaller. The efficiency of TTOs that were below the average efficiency score in 2011, in this case #14 and #29, was greatly improved in 2015. 11 In contrast, it is difficult to find a systematic difference between 2011 and 2015 for the consortium group. The plot shows that the relative efficiency of TTOs in the consortium group was exacerbated, or at least was not improved. Interestingly, the unsupported group improved in terms of both outcome and efficiency.
More technology transfer contracts and greater amounts of royalty income are commonly the major objectives of TTOs, but each TTO may assign different weights to these goals depending on the strategies they employ. TTOs with high-value technologies may try to focus on transferring technologies at higher prices. On the other hand, we can conjecture that the majority of TTOs may try to increase their overall revenue by signing more contracts. Figure 4 shows a scatter plot of the number of technology transfers contracts against royalty income. The two variables are positively correlated12 but the data points are more dispersed with an increase in either the number of contracts or royalty income. This reflects the possibility that the behavior of the TTO becomes diversified as the TTO’s capacity increases. Therefore, it is worthwhile to estimate our model with the number of technology transfers contracts as the dependent variable.
Table 5 presents the coefficient estimates and Table 6 provides the associated marginal effects of the inefficiency determinants. We compare the results with the estimates in Section III-C. Regarding the estimation of the Frontier Equation, the coefficients of TTO employment and domestic patents are estimated to be similar to the previous results. However, the sign of the foreign patent coefficient is estimated to be negative and significant. This is an interesting result in that it implies that when more foreign patents are owned, fewer technology transfers occur. This may be in line with our conjecture that TTOs with potentially high-value technologies may seek to focus on raising the value of their technology rather than on the quantity of their contracts.
The most noticeable difference between the inefficiency equation estimation results shown in Table 5 against Table 3 is that the magnitude of the overall coefficient estimates is reduced. In addition, the signs of the public university dummy become negative and the standard errors shrink, even if we cannot reject from specifications (A5) – (A7), showing that R&D funding does not have significant effects on the inefficiency distribution, whereas an increase in total financial support helps reduce the inefficiency of TTOs. The marginal effects of the inefficiency determinants are also estimated to be comparable with slightly decreased magnitudes, as displayed in Table 6.
Korea's R&D investment is steadily increasing, along with the share of government and public resources. In the early 2000s, the Korean government began to recognize the importance of technology transfers and put forward policies to promote them. The Leading TTO Cultivation Project is one of these technology transfer promotion policies, which is relevant in that it directly supports technology transfer intermediaries. This policy has had a positive effect in that TTOs and related experts at universities have expanded quantitatively and the interest in technology transfers has increased.
This study empirically analyzed the effects of exogenous variations, in this case the research capacity, amounts of funding and financial aid, and the university type, as well as policy interventions, on the operational efficiency of TTOs. We use a production function approach, in particular a stochastic frontier analysis, to estimate the efficiency scores for each TTO and the marginal effects of exogenous variables. Our empirical results suggest that the smaller the research capacity and the amount of financial aid for the university to which the TTO belongs, the larger the marginal effects of exogenous variables. More importantly, the marginal effect of the Leading TTO Cultivation Project was not monotonic and was greater for the TTO group which was supported as a type of consortium than for the leader group. The policy originally aimed to support TTOs which showed excellent performance initially, followed by help for the growth of late-runner TTOs. Our results imply that the policy goal is not fully achieved in that the effects on the target group did not outperform the effects on the other group. Nevertheless, the implementation of the project helped to reduce inefficiency on average. This result is unchanged when we estimate the model with the number of technology transfer contracts as a dependent variable.
Therefore, we conclude that the policy may have been more effective if a detailed policy design had been provided considering the different conditions of TTOs. If more subsidies are provided to late-runners and non-monetary support such as technology promotions and the easing of legal constraints could be provided to the front-runners, the policy effect may be maximized. In addition, the policy effect can be maximized if different policy devices are implemented considering each university's research capacity, financial environment, and the characteristics of the TTOs.
As noted above, there are more policies for the purpose of vitalizing industry-university cooperation and the growth of TTOs than the Leading TTO Cultivation Project analyzed in this study. Therefore, it is difficult to conclude that the government policy is too monotonous based solely on the analysis conducted here. Nevertheless, this study has significance in that it quantitatively assessed the effectiveness of the TTO operating-cost support policy and pointed out that this policy could be further improved.
In addition, it is important to point out that the direction of the government's TTO support policy must be clearly defined. The Leading TTO Cultivation Project aims to support TTOs already equipped with technology transfer capabilities to reach a higher level of TTO. However, as shown in this study, the policy effects associated with efficiency improvements were greater in the second-tier group than in the leader group and were larger in relatively small colleges and in those that lack capacity. This confirms that there is a gap between the current policy goals and the means by which to realize them. If the government wants to maintain its current goal of increasing the growth of leading TTOs, the size of the subsidy should be increased to match the size and capacity of the school. However, if the government wants gradually to reduce support for leading TTOs and enhance support for latecomer TTOs, the current criteria for the selection of support colleges should be changed.
Finally, it should be noted that the analysis in this paper does not consider the production of research outcomes. In other words, even when the research production performance of a school is poor and the outcome is not continuously produced, the school is classified as highly efficient if the technology transfer performance against input is excellent. This case was not addressed separately, but the desirable policy direction for such cases would be to secure the mobility of skilled technical transfer personnel to other schools and to provide support for research capacity improvements of such colleges.
Note: [1]: No. of technology transfers, [2]: royalty income, [3]: No. of TTO staff members, [4]: operational expenses, [5]: government research funding, [6]: total research funding, [7]: total financial support, [8]: No. of students, [9]: No. of faculty members, [10]: total education expenses, [11]: domestic patents granted, [12]: foreign patents granted.
In Korea, several laws were enacted or revised by benchmarking the Bayh–Dole act. Three representative acts are the Technology Transfer Promotion Act (enacted in 2000), the Industry Education Enhancement and Industry—Academia—Research Cooperation Promotion Act (amended in 2003; IARC Promotion Act hereafter), and the Invention Promotion Act (amended in 2006). The enactment of TTPA made it possible to manage intellectual property through TTOs, but this law encompasses not only universities but also the TTOs of government research institutions. Meanwhile, the amendment of the IARC Promotion Act in 2003 made it possible for the Industry-Academia Cooperation Foundation to acquire legal status and handle integrated issues such as intellectual property rights management, affairs of technology transfers, and researcher compensation. The amendment of the Invention Promotion Act in 2006 allowed teaching staff of a national or public school to have a non-exclusive license for employee invention. Accordingly, detailed policy devices related to the laws originated from the spirit of the Bayh–Dole act, and some of the policy programs implemented according to each law may resemble each other. For example, the Leaders in Industry-University Cooperation + (LINC+) project is similar to but more comprehensive than the Leading TTO Cultivation Project. In this paper, we focus on the policy effects of the Leading TTO Cultivation Project and our results are therefore limited in that we cannot address all of the complicated relationships between policies.
On the basis of TTPA, the government mandated the establishment of technology transfer offices (TTOs) at national and public universities and encouraged technology transfer activities.
The Science and Technology Innovation Office is a subordinate organization of the Ministry of Science and Technology. It is responsible for the coordination of the related departments of S&T policies, industry, manpower, and regional innovation policies, along with the allocation of the R&D budget and performance evaluations.
The relative inefficiency term can be transformed into the relative efficiency measure through a negative exponential function. Hence, efficiency and inefficiency terms are used interchangeably in this paper.
A one-sided distribution of inefficiency can be modeled in various ways. The widely used distributional forms are the truncated normal, half normal, exponential, and gamma distribution.
Wang (2002) also provided the functional form of the marginal effects of determinant on the variance of efficiency. We do not present the functional form here, as our main interest is on the location rather than the dispersion of the efficiency distribution.
The operating expenses for university–industry cooperation do not include remuneration for TTO employees. Therefore, there is no problem with duplicated calculations between the number of TTO staff members and labor costs in the selection of input factors.
There were 408 institutions of higher education in 2007, and this number increased to 431 in 2015.
The determinants of the probit model are total research funding, the number of TTO staff members, domestic and foreign patent amounts, operational expenses, total financial support, and the number of faculty members.
, & (1995). A Model for Technical Inefficiency Effects in a Stochastic Frontier Production Function for Panel Data. Empirical Economics, 20(2), 325-332, https://doi.org/10.1007/BF01205442.
(2000). Technology Transfer and Public Policy: A Review of Research and Theory. Research Policy, 29, 627-655, https://doi.org/10.1016/S0048-7333(99)00093-1.
, , & (2015). The Evolving State-of-art in Technology Transfer Research: Revisiting the Contingent Effectiveness Model. Research Policy, 44, 34-49, https://doi.org/10.1016/j.respol.2014.06.008.
, & (2010). Performance of Spanish Universities in Technology Transfer: An Empirical Analysis. Research Policy, 39, 1160-1173, https://doi.org/10.1016/j.respol.2010.05.016.
, & (2002). Technology Transfer in United states universities. Journal of Evolutionary Economics, 12, 199-232, https://doi.org/10.1007/s00191-002-0105-0.
, & (1993). Biases in Frontier Estimation due to Heteroscedasticity. Economic Letters, 41(1), 17-20, https://doi.org/10.1016/0165-1765(93)90104-K.
, & (2003). University Technology Transfer: Do Incentives, Management, and Location Matter? The Journal of Technology Transfer, 28, 17-30, https://doi.org/10.1023/A:1021674618658.
, & (2003). Bottom-up versus Top-down Policies towards the Commercialization of University Intellectual Property. Research Policy, 32, 639-658, https://doi.org/10.1016/S0048-7333(02)00034-3.
, & (1994). Estimation of a Non-neutral Stochastic Frontier Production Function. Journal of Productivity Analysis, 5, 171-180, https://doi.org/10.1007/BF01073853.
, , & (2007). Technology Transfer Offices and Commercialization of University Intellectual Property: Performance and Policy Implications. Oxford Reviews of Economic Policy, 23(4), 640-660, https://doi.org/10.1093/oxrep/grm036.
, , & (2003). Assessing the Impact of Organizational Practices on the Relative Productivity of University Technology Transfer Offices: An Exploratory Study. Research Policy, 32, 27-48, https://doi.org/10.1016/S0048-7333(01)00196-2.
, & (2002). Growth and Productive Efficiency of University Intellectual Property Licensing. Research Policy, 31, 109-124, https://doi.org/10.1016/S0048-7333(00)00160-8.
, & (2002). Who is selling the Ivory Tower? Sources of Growth in University Licensing. Management Science, 48(1), 90-104, https://doi.org/10.1287/mnsc.48.1.90.14271.
(2002). Heteroscedasticity and Non-monotonic Efficiency Effects of a Stochastic Frontier Model. Journal of Productivity Analysis, 18(3), 241-253, https://doi.org/10.1023/A:1020638827640.
, & (2002). One-step and Two-step Estimation of the Effects of Exogenous Variables on Technical Efficiency Levels. Journal of Productivity Analysis, 18(2), 129-144, https://doi.org/10.1023/A:1016565719882.